Incremental Delta Copying from SQL Server to Apache Iceberg
Learn how you can load data from SQL Server to Apache Iceberg in an incremental way.
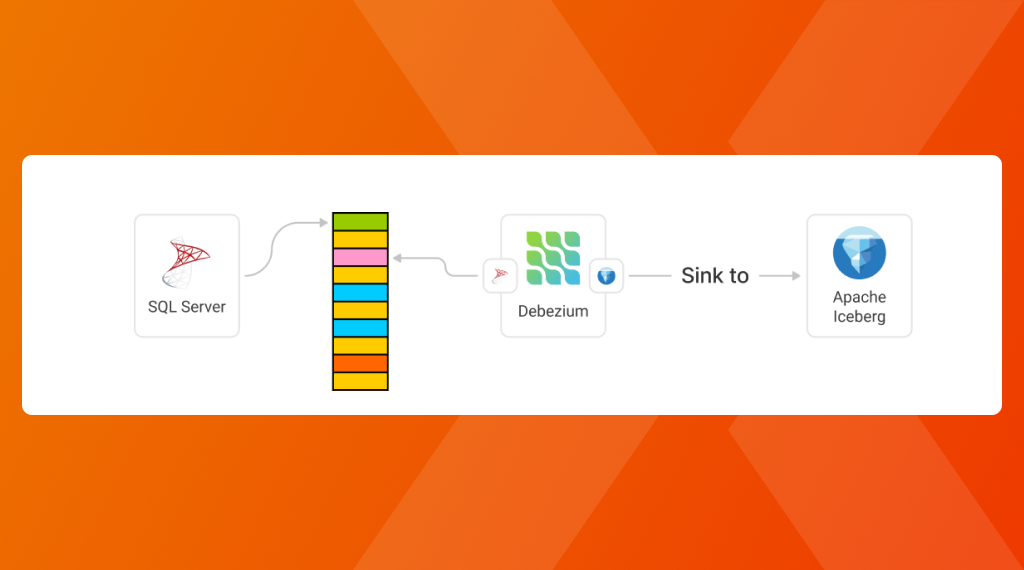
Are you looking to implement Business Intelligence (BI) in your organization but unsure where to begin? For many companies, SQL Server serves as the primary database, making it tempting to simply connect your BI tools directly and start generating reports. While this approach seems straightforward, it’s often not the best solution. Running BI reports directly on your application database can create significant load, as these databases aren’t typically optimized for BI queries.
Running BI reports directly on your application database can create significant load, as these databases aren’t typically optimized for BI queries.
So, what’s a better alternative? Enter the “Flat File” approach! Flat files have come a long way—from being slow and cumbersome to becoming scalable and efficient, thanks to modern technologies like Apache Spark and, more recently, Ray with Daft. Leveraging flat files offers several advantages:
- Reduces load on your main application database
- Provides greater flexibility
- Enables additional ETL steps, such as removing sensitive (PII) data
Of course, while the benefits are clear, implementation requires careful planning. Rather than simply dumping all your data into a flat file, it’s important to consider how you load data—specifically, how to do this incrementally instead of performing full loads every time, which can quickly become inefficient.
In this article, I’ll guide you step-by-step on how to set up an incremental data pipeline from SQL Server to Apache Iceberg.
Existing Tools
As for everything, there are a lot of tools available for the job. However, after researching the most popular ones, I came across just a handful ones, making this a very difficult problem to solve!
I came across just a handful ones, making this a very difficult problem to solve!
Let's compare some of them:
- Airbyte: Amazing ETL tool, batch only, but manages the CDC for you and you are able to self-host it (although it being a complex tool)
- SQL Server CDC: SQL Server provides CDC natively, so we can build custom scripts (cumbersome) to do this.
- Estuary Flow: A lot-based replication tool, which does the trick, but is very expensive for lakehouse purposes.
- Sling: CLI Tool for data loading, which requires more work as you are required to define the keys or SQL Queries for the diffs.
- DLT Hub: Another ETL tool comparable to Sling, but most of the CDC features it has are behind dlt+ (paywall)
- Debezium: Has been along for a long time, and is quite complex through its Kafka consumer. HOWEVER! A Debezium Server for Iceberg was created, making this an amazing solution.
Leading me to concluding that either Airbyte or Debezium are the most cost-efficient and future-proof solutions!
Architecture
Looking at the architecture, we will then have SQL Server that writes the latest changes to its LOG file, whereafter we have Debezium monitoring this log and replicating it to our Apache Iceberg installation.
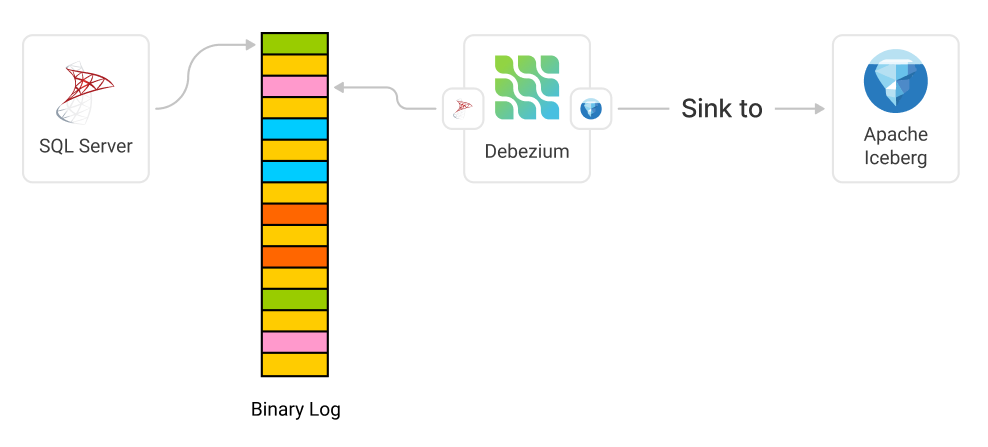
Configuring Debezium for SQL Server to Iceberg
Prerequisites
- A SQL Server connection with all the details
Getting Started
Setting this up, we create a Docker Compose application (based on the Debezium Iceberg Server example), with the SQL Server source configured in the application.properties
file:
# #######################################################
# ############ DEBEZIUM SOURCE CONFIGURATION ############
# #######################################################
# SQL Server Source
# (https://debezium.io/documentation/reference/stable/connectors/sqlserver.html#sqlserver-example-configuration)
debezium.source.connector.class=io.debezium.connector.sqlserver.SqlServerConnector
debezium.source.offset.flush.interval.ms=0
debezium.source.database.hostname=your-hostname-here
debezium.source.database.port=1433
debezium.source.database.user=main
debezium.source.database.password=your-password-here
debezium.source.database.names=main
debezium.source.topic.prefix=dbz_
# saving debezium state data to destination, iceberg tables
# see https://debezium.io/documentation/reference/stable/development/engine.html#advanced-consuming
debezium.source.offset.storage=io.debezium.server.iceberg.offset.IcebergOffsetBackingStore
debezium.source.offset.storage.iceberg.table-name=debezium_offset_storage_table
# see https://debezium.io/documentation/reference/stable/development/engine.html#database-history-properties
debezium.source.schema.history.internal=io.debezium.server.iceberg.history.IcebergSchemaHistory
debezium.source.schema.history.internal.iceberg.table-name=debezium_database_history_storage_table
Once this is configured, we can spin up our instance with:
docker-compose up -d
# Load the Notebook Server
http://localhost:8888
# Extra URLs
http://localhost:8080 # Trino
http://localhost:9000 # MinIO
http://localhost:9001 # MinIO UI
http://localhost:5432 # PostgreSQL
http://localhost:8000 # Iceberg REST Catalog
Demo
Seeing this in action, we can see a proper incremental data load mechanism, retrieving the latest data from our SQL Server and copying this over to our sink (apache iceberg).
Comments ()